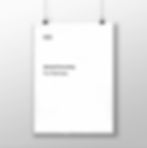
The team at Remi AI has written about demand forecasting on several occasions in different contexts. This blog post will bring a few key concepts together in the context of the business lifecycle. Having delivered forecasting solutions for companies both large and small, the team at Remi AI has pulled together some thoughts on the forecasting problems experienced at each stage, as well as some solutions that we have implemented to overcome these.
For reference, we will discuss the classic business life cycle taught in every business school classroom and textbook on the planet, shown below:

We will break this out into four pieces that cover the major stages of business, and as usual will update the below links as we produce each piece:
Start Up
Growth
Maturity
Decline
Demand Forecasting for start-ups
The first piece of information to communicate is that start-ups will likely not have enough data to make many time series forecasting approaches feasible. The concept of “enough data” varies from business to business, though we can’t stress enough the importance of start-ups capturing data from day 1. Whether that be Google Analytics, or via the ERP, data will be a key building block for the future.
For anyone familiar with A.I and data science, you will understand that not having enough data is a particularly prickly problem. A.I models, as well as the majority of more standard statistical methods, require historical data to build an understanding of the demand profile before a forecast can be made, the more the better.
So, without that, how does one forecast the future without any clue as to how the demand profiles will behave?
Good question.
Firstly, forecasting with no historical data is never perfect (more art than science), though there are approaches that can at the very least provide some contextual information.
The first useful approach to communicate here is called clustering — we have written about this previously here. Essentially, clustering is used to group together data points that are more similar in some dimensions than other data points in the data set. This can be understood using a handy visual aid thusly:

So, armed with a clustering, we are then able to utilise the time series of similar products to predict those of start-up products. As with almost all forecasting, a human layer is useful to sense check the results. We can never claim that this works perfectly (no forecasting does), but it does provide another lens to assist startups with their planning.
The second concept to communicate is around neural networks. This is not a new concept (the idea has been around for decades), though some recent time series architectures allow a forecasting engine to be trained up on many different time series’ (e.g. 500 products), and for the network to then forecast a new product.
Sound crazy? You bet — we can never claim that the results are perfect for this, though it can go a long way to granting a startup information on their future sales that they otherwise would not have.
The Remi AI forecasting suite includes both methods above and we have helped several startups with their forecasting needs.
The final point to raise is the more traditional forecasting approach for start-ups involving top-down and bottom-up analysis. These techniques are in use across financial markets and can again prove useful in providing context to a start-up owner looking to build an idea of upcoming sales.
Option 1: Top Down Analysis:
Starting your assessment at a macro level to find market size and potential market growth, and then estimating your own revenue as a function of your assumed market share.
Step 1: Estimate market size
Step 2: Estimate market growth
Step 3: Estimate your market share at some point in future
Step 4: Calculate the corresponding revenue
Option 2: Bottom Up Analysis:
Multiplying the number of likely sales for each product, the average value of these sales, when they are likely to occur. Building in a growth rate allows you to view this through time.
The above is not a time series approach and so will only provide an output for a specific point in time. The analyses are also extremely sensitive to the inputs such that under/overestimating any one of the inputs can mean that the results are severely affected.
What’s Next for AI and Inventory Management?
Our humble goal is to revolutionise supply chain management. While working towards solving the different challenges associated with demand forecasting at different stages of business lifecycle, we are simultaneously working to improve delivery scheduling, warehouse simulation, and global logistics simulation into our platform.
The advantage of an entire supply chain being managed by the same AI platform is the benefits reaped from these different components speaking to each other. It truly is a hugely exciting time to be working in this space.
Stay tuned on the Remi AI blog as we build out the complete supply chain offering!
Or, if you’re ready to start seeing the benefits of A.I-powered inventory management, start the journey here.