When most of us hear the phrase “Automotive A.I” it’d be a safe bet that the first item to pop into our heads is one of self driving cars. Who could blame us, when there are some extraordinary companies out there making headlines every week (both good and bad) in this domain, such as Uber, Zoox, and Tesla. For those who have had their fill of these headlines, fear not, this article is not about that particular intersection of the automotive world and Artificial Intelligence. Instead, I would like to focus on an easily overlooked area of the industry; Supply Chain and Replenishment.
Starting with Supply Chain, there are three broad areas that stand to benefit most from A.I;
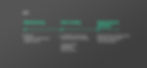
Automotive Manufacturing:
Firstly: Robotics. The GIF below shows a Tesla robotics factory (Again, I promise that I won’t mention autonomous driving here). The level of automation in this factory is hyper-advanced, with nothing coming close to the scale of this that this particular author knows of. Its development has not come without issues, however, with a very public about-face on the efficacy of robots versus human workers from Elon Musk in 2018 (more on that here). Even so, robotics, which can incorporate multiple domains of A.I such as computer vision, reinforcement learning, and natural language processing, has the potential to add significant value to the automotive manufacturing process through time and cost saving.
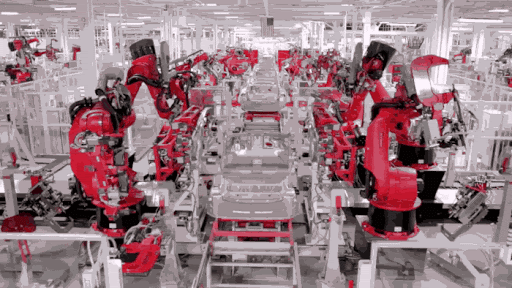
The good people at McKinsey and Company put out a research report in 2017 (read that here) which suggested that a holistic approach will be required to increase the chances of a successful implementation. Such an approach requires executives to put a project in the context of their business and strategy, allowing a major technology initiative such as a robotic factory to “align closely with the current and future needs of the business” - to avoid a feeling amongst the general staff of tech for the sake of tech. We agree wholeheartedly with this, and think that this advice can be transplanted to many other technology transformation domains. The McKinsey article also shows the decreasing cost of robotics, making scenes like the one above more and more available to smaller manufacturers.
Secondly, Predictive Maintenance. Whilst not a new concept, the advent of mainstream A.I combined with better quality machine data (thanks largely to IOT) has seen an improvement in the methods with which companies can predict machine failure. We wrote a blog post on one such method early in 2019 which can be found here for reference, so we won’t rehash that here.
We will instead discuss some of the challenges that must be overcome for a successful predictive maintenance implementation.
Data quality: as with almost all A.I approaches, the Garbage In Garbage Out (GIGO) effect applies. When asking an A.I to produce an output such as a prediction of machine failure, if the data is of poor quality, it is folly to expect better than poor results. There are ways of managing this via feature engineering and early analysis (though this does increase the project workload and therefore cost).
Integrations: consider the case of an automotive manufacturer wishing to predict when its machinery may break down. One way to attack this problem is to analyse live machine data to assess the likelihood of a failure ‘event’ occurring at any given point, prompting a preventative action to be taken once the likelihood of a failure event passes a threshold. Such an approach would potentially require integrations with many systems, ranging from ERPs, WMSs, CRMs, and any specific machine software. These integrations typically add a significant amount of time to the project and must be planned for.
Change Management: “I’m supposed to trust a new piece of technology that is telling me that there is a 95% chance that my machine will fail soon, rather than following my tried and tested preventative maintenance schedule?”. If your answer to this question is “yes, and don’t ask any more questions”, your predictive maintenance endeavour is on a path to certain doom. Your people are the ones who will act on your shiny new predictive maintenance technology, and must be brought along for the journey. Collective buy-in is imperative for ongoing success.
Finally, to Quality Control. Computer vision has advanced greatly in the 30 years since the foundational research paper on convolutional neural networks by Yann LeCun in 1989. The domain is advanced to the point that a conveyer belt or large machined panel can be scanned by a camera, analysed for defects by a computer vision method, and accepted or rejected based on the result. What was once a repetitive job for humans, can now be done with impressive accuracy and at a fraction of the cost.
Data Tracking:
The second domain of interest for automotive A.I centres around data tracking, and the previously unused datasets that are becoming available to businesses today.
Firstly, the previous section introduced the use of computer vision in quality systems, and this can be extended to include analysis of movement through space. Consider an automotive retailer with hundreds of locations around a nation. Such a wide network could facilitate experimentation in store layout using data collected via computer vision methods. Using relatively simple object detection (e.g. a YOLO Network), an understanding of customer behaviour can be built to include insights such as:
Length of time spent at each shelf
Path through the store
Time taken until first item found
Using the above, retailers can optimise the designs of their stores to suit their goals, something that would have been far more difficult without this data.

Secondly, language data in customer interactions such as customer service calls is likely an untapped resource for many businesses in the automotive world. If you are in automotive sales, chances are you have plenty of back and forth with customers preparing quotes and negotiating. Consider a classification engine that could predict the likelihood that a deal will close based only on the chat transcript of your sales calls. This would no doubt prove to be a useful tool for those in sales looking to streamline their time, devoting the most energy to the opportunities most likely to convert (or the obverse if you’re competitive?). Analyses of this nature would again not be possible without a Natural Language Processing and classification system that could transcribe speech to text, before making a prediction on the likelihood of the conversion.
Optimisations and Decisions:
The final, and in our opinion the most exciting, domain of A.I available to automotive businesses is that which deals with optimisations and decisions. The previous sections have largely dealt with a domain of A.I called Supervised Learning which entails an A.I looking at a large labelled dataset, learning the patterns (training), before being shown an unlabelled example and making a prediction/classification of what the A.I thinks the label should be. A separate branch of A.I called Reinforcement Learning (RL) is advanced to the point that it can act on the world around it. This is A.I that learns to operate in an environment, and is rewarded and punished based on what it does (for an introduction to Supervised, Unsupervised and Reinforcement Learning, read here). Reinforcement Learning is best illustrated with an example rather than conceptual preaching; consider the decision domains below.
Firstly, inventory replenishment. We have written about this topic at length here, so please feel free to review at your leisure. I will summarise the points relevant to automotive retail in this post. Consider the automotive parts retailer mentioned above which has 300 stores across a nation. Chances are that the demand profile of each SKU behaves differently, and even the demand of the same SKU in different locations (store_item_pair) is likely to be unique. Each store is likely to have a different amount of space in the storeroom, potentially a different inventory budget, and even a different product mix. These differing dynamics can be simulated using historical data, and an RL agent can then be placed into the simulation, given a goal e.g. keep stock availability above ABC% with a maximum working capital budget of $XYZ, and allowed to find the ideal purchasing policy at each location.
To illustrate this, the image below shows inventory levels of 1 SKU for a period of 12 months. An RL agent was placed into a simulation of the purchasing environment for this SKU, and allowed to explore different purchasing policies while optimising for stock availability and working capital. The blue line indicates the ACTUAL inventory levels that the business experienced using their previous replenishment process. The green line represents the OPTIMISED inventory holding that would have been experienced had the RL agent been in control of replenishment during this period. The inventory levels were held at a much more reasonable level for this SKU using the optimised purchasing policy when compared with the actual data, potentially saving the business thousands of dollars in working capital.

For further information on how A.I can be used in Inventory Management please feel free to check out this link (and excuse the shameless plug).
Secondly, allocation optimisation. When an automotive retailer has a limited amount of new inventory, a key question is how best to distribute that throughout the network. The decisions made at this point in a retailer’s process has the potential to significantly impact the bottom line, with lower performing locations potentially overstocked and higher performing stores understocked. A.I allocation can provide the missing link in the chain for an automotive retailer looking to distribute inventory to the greatest effect throughout their network. A.I systems can ingest, combine, and analyse billions of data points every day to ascertain where best to distribute inventory within a store network.
Finally, price optimisation. While not a part of the supply chain, pricing decisions can have a marked impact on demand, and given that almost all the activities mentioned above (manufacturing, replenishment, and allocation) are driven by demand, how could we not tie it all together with a customer facing demand driver?
In much the same way that RL is applied to inventory replenishment, it can also be applied to pricing decisions. An A.I system can forecast the likely demand at different price points on the price elasticity of demand (PED) curve (example from the literature below for anyone keen to brush up on their microeconomics). Put simply, the thinking goes that products have different price elasticities, meaning that their demand is affected more/less to changing prices depending how steep their PED curve is.

Using historical data and some price exploration, an A.I system can build its own PED curve for each SKU, combine this with external data streams such as competitor prices, and programmatically set website prices for an ecommerce automotive business. The A.I can be given a goal such as “grow the top line”, and it will try to do so within the business constraints built in such as:
Never go below 110% of cost price
Never be the most expensive in the market
Again, the good people at McKinsey wrote a fantastic report on Price Optimization in ecommerce (link here) where they suggest that, using an inclusive approach, retailers can:
“...capture significant and sustained impact—typically, sales growth of 2 to 5 percent and increases of 5 to 10 percent in margins, along with higher levels of customer satisfaction through improved price perception on the most competitive items.”
You’ll notice that all of the examples above are centred around a goal of capital efficiency. RL can be a fantastic addition to the toolkit of an automotive business looking to drive efficiency across data heavy decision domains.
To bring this novel to a close, I’ll conclude by saying that A.I has enormous potential in the automotive industry. The three domains mentioned here; manufacturing, data tracking, and optimisations, are no doubt just the beginning. Any forward thinking business leaders working in the automotive industry who would like to explore what is possible in your business, please feel free to reach out for a confidential discussion.
Eamonn Barrett is a founder at Remi AI. He loves all things A.I, is a Level 3 CFA Candidate, lives in Sydney and is the proud owner of the company's second best moustache.