The Importance of Demand Forecasting
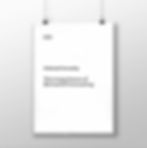
This article is the second in a series on how Remi AI deploys forecasting to help clients improve their operations, increase revenues, reduce wastage and find optimisation opportunities. In recent years, deep learning, probabilistic programming and machine learning have delivered significant improvements on traditional time series methods in generating accurate forecasts. Leveraging these three new approaches in addition to the more traditional statistical methods, Remi AI builds solutions for clients across a range of problems.
The need for Forecasting is ever-growing. Previously it was mostly utilised for revenue and short-term supply chain, but in recent times companies across most industries have been deploying forecasting across predicting production, revenue, staff utilisation, web traffic, call centre utilisation, vehicles needed, goods to be ordered and number of employees needed to maintain growth.
At Remi AI, we’ve deployed forecasting for a wide variety of organisations, from small E-Commerce to numerous Fortune 100 Companies. Some interesting examples include:
Supply Chain Forecasting.
A critical and staple deployment of forecasting for us. Working with our clients, our platform currently makes daily forecasts for over 100 million products across the United States, United Kingdom and Australia. We use it to predict both Supply and Demand at a granular level and at upper company levels. Allowing our customers to make informed strategic replenishment decisions and to automate their supply chain with automated replenishment AI. If you’re a developer and interested in utilising machine learning for your forecasting, you can leverage our APIs.
Stadium Attendance.
Staffing, food and beverage planning for stadiums is a complex and costly problem. Under provisioning leads to large queues, reduced sales and complaints. But over-provisioning can be costly — especially with inventory with a short lifespan like food. Our forecasting has delivered attendance estimates with over 98% accuracy, allowing the planners to accurately provision for the event.
Spatio-Temporal Forecasting.

Although spatio-temporal forecasting is still primarily an open area of research, we have delivered forecasting for both user demand and user destinations for Mobile Apps. This predicts user demand across spatio-temporal dimensions, allowing our customers to understand upcoming demand and optimise their delivery/pick up planning.
How has forecasting changed recently?
In the last few years, the machine learning industry has developed a number of new methods that are often outperforming the mainstream statistical approaches that have dominated time-series forecasting for the last decade. Many of these new approaches not only allow you to ingest many different variables (which is already an advantageous advancement over more traditional time-series forecasting) but some of the new methods also allow you to ingest your future plans into your demand forecasting, directly into the model. Future-planned promotions and pricing changes can now be pulled directly into the model.
At Remi AI, ensuring we choose the right forecasting method for the many different use cases involves answering the following key questions:
1. Is the product high velocity or low velocity? I.e. is the product selling many each day or does it only sell one once a week.
2. Does external data (such as Weather, Exchange Rates, Web Traffic) have a role to play in the forecast?
3. Is it a new product that has little or no historical sales data?
With this in mind we will choose the most suitable subset of models for the product.
But what if you’re using our Demand Forecasting APIs and are not sure what the answers are for the above questions? Or if you simply have too many products and it would take too much time to isolate each product off? You can simply use the AutoML function in the APIs. AutoML (Autonomous Machine Learning) utilises Machine Learning to choose the best model, hyper-parameter optimisation, and feature Engineering. The trade off is it takes longer, because the AutoML needs more time to test and settle on the best model, but it allows you to get on with everything else.
If you’d like to learn more about Demand Forecasting and how it can help your business, please let us know.
Or if you like to just jump into the APIs yourself, then signup!